
IEEE 802.11, known as Wi-Fi, has become the main standard for wireless local area networks. Wireless networks have become one of the most important ways to connect devices to the Internet, therein improving productivity and encouraging information sharing. This increase is related to advances in wireless data communication technologies. Technical reports indicate that wireless and mobile devices will account for 71% of all IP traffic by 2022, an increase of 19% over four years. By identifying the different error sources and analyzing their determinants, we recommend (4) identification strategies for future research.
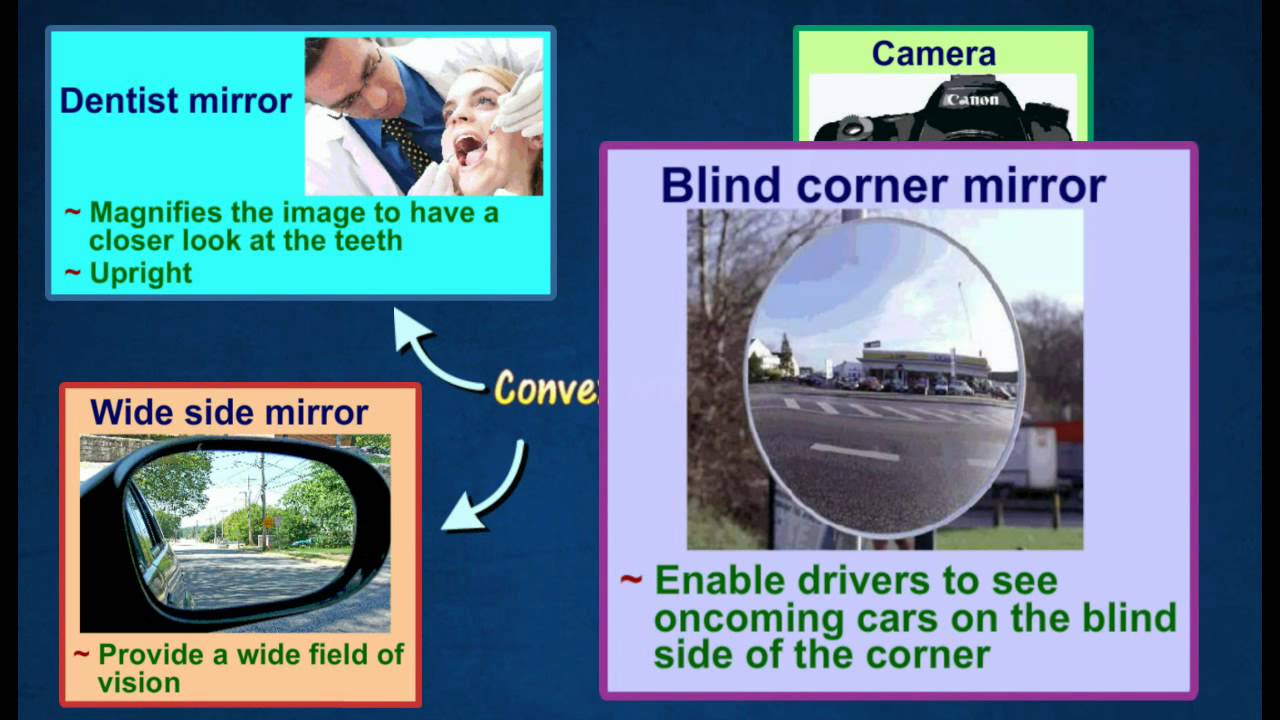
We (3) raise awareness of error sources in geolocation measurement, such as the use of GPS falsifier apps, or device sharing among participants. To disentangle the different influences, we (2) apply a multistage error model to analyze and control the error sources in the specific missingness process of geolocation data. Instead, device-related error sources, such as the manufacturer and operating system settings, design decisions of the research app, third-party apps, and the participant, can interfere with the measurement. In our article, we demonstrate (1) that sensor data are far from error-free. Our findings can be applied to the wider subject of sensor data. Geolocation sensor data are an example of an available data type that is of interest to social science research. Using data from a 2018 German population-based probability app study, this article focuses on the measurement quality of geolocation sensor data, with a strong focus on missing measurements. However, little is known about the quality of the resulting data.
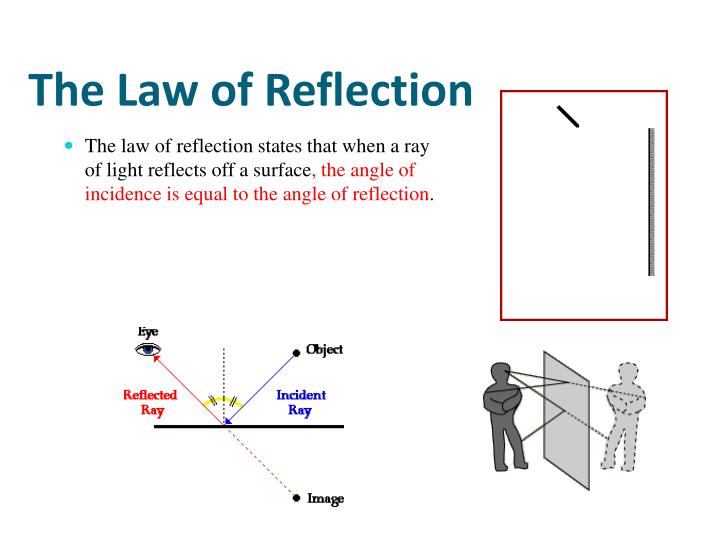
Information on day-to-day geolocation could provide valuable insights into human behavior that cannot be collected via surveys.
Reflection refraction diffraction free#
Passively collected data from sensors that measure geolocation or movement provide an unobtrusive way to observe participants in everyday situations and are free from reactivity biases. Moreover, it is found that the integration of Angle‐of‐Arrival measurements in particle‐filter‐based positioning improves the 3‐D positioning accuracy by about 37.3% on average.Īs smartphones become increasingly prevalent, social scientists are recognizing the ubiquitous data generated by the sensors built into these devices as an innovative data source. Compared with the standard particle filter, the particle filters with our proposed approaches achieve similar positioning accuracies with less computation time. The test result shows that the particle filter with the proposed approaches performs similarly to the Rao‐Blackwellized particle filter and slightly better than the particle filter with IMU. They are integrated into particle filtering algorithms for prior position determination.

This paper proposes two novel velocity determination approaches, which use measurements to approximate velocity in a self‐contained way. Both methods have limitations when using them in practice. Velocity can be obtained from an inertial measurement unit (IMU) sensor or the posterior state vector at the previous time stamp.

Filtering‐based indoor positioning using ultra‐wideband (UWB) requires known velocity to predict prior position in the prediction stage.
